Future of AI in medical imaging: Challenges and opportunities
The intersection of artificial intelligence (AI) and medical imaging has become a strategic area of focus for researchers, healthcare professionals, and academics alike. As medical imaging technologies advance, the volume and complexity of data generated by CT scans, MRIs, and endoscopies are beginning to outpace traditional diagnostic tools. Radiologists and healthcare providers now face the daunting task of managing and interpreting this vast amount of data in a way that genuinely enhances patient care. This is where AI can make a real difference—by improving diagnostic accuracy, automating repetitive tasks, and offering new insights that were once out of reach.
However, incorporating AI into medical imaging is not without its challenges. With various imaging techniques posing their own set of hurdles, solutions that can be adapted to different clinical settings are needed. Existing systems still require refinement to improve usability, speed, and accuracy, and researchers are leading the way in this area. They are working to overcome the limitations of current medical imaging practices and envision a future where AI and personalized medicine are key players in enhancing diagnostics and patient care.
AI’s role in medical imaging is expanding beyond assisting with routine tasks. The future of AI in medical imaging holds the potential to completely transform how we detect diseases like cancer, interpret medical images, and even predict patient outcomes. As AI algorithms continue to improve, they can autonomously analyze medical scans with greater speed and precision, reshaping the role of radiologists. Far from replacing them, AI will act as an invaluable tool, enhancing their capabilities and allowing them to focus on more complex cases and innovative problem-solving.
Use of AI in medical imaging
Artificial intelligence rapidly transforms medical imaging, changing how healthcare professionals approach diagnostics. Through machine learning and robotics, AI enhances the efficiency and accuracy of medical imaging, providing powerful tools for clinicians. Central to this shift is machine learning, a subset of AI that trains algorithms to recognize patterns and abnormalities in imaging data, often detecting issues that might be missed by the human eye.
Standard imaging methods like CT scans, MRIs, X-rays, and endoscopies produce vast amounts of visual data, which can be overwhelming for clinicians to analyze manually. AI algorithms help by processing these images and highlighting critical patterns and potential anomalies vital for accurate diagnosis. In practice, AI can draw attention to areas that require further scrutiny, suggest possible diagnoses, and even automate time-consuming tasks such as scheduling imaging studies or optimizing equipment usage. This automation streamlines workflows and reduces human error—especially given that research suggests up to 4% of diagnostic interpretations may contain clinically significant mistakes.
Furthermore, AI can integrate and analyze data from various imaging modalities, enabling clinicians to interpret complex, multimodal information. For example, AI might combine CT scan images with genomic or pathology data to offer a more comprehensive understanding of a patient’s condition—something that would be difficult to process quickly without AI’s assistance. As AI systems continue to evolve, they are expected to enhance radiologists’ abilities further, allowing them to tackle more complex cases while automating repetitive tasks currently dominating their workflows. This partnership between AI and human expertise is key to achieving more efficient, precise, patient-centered diagnostics.
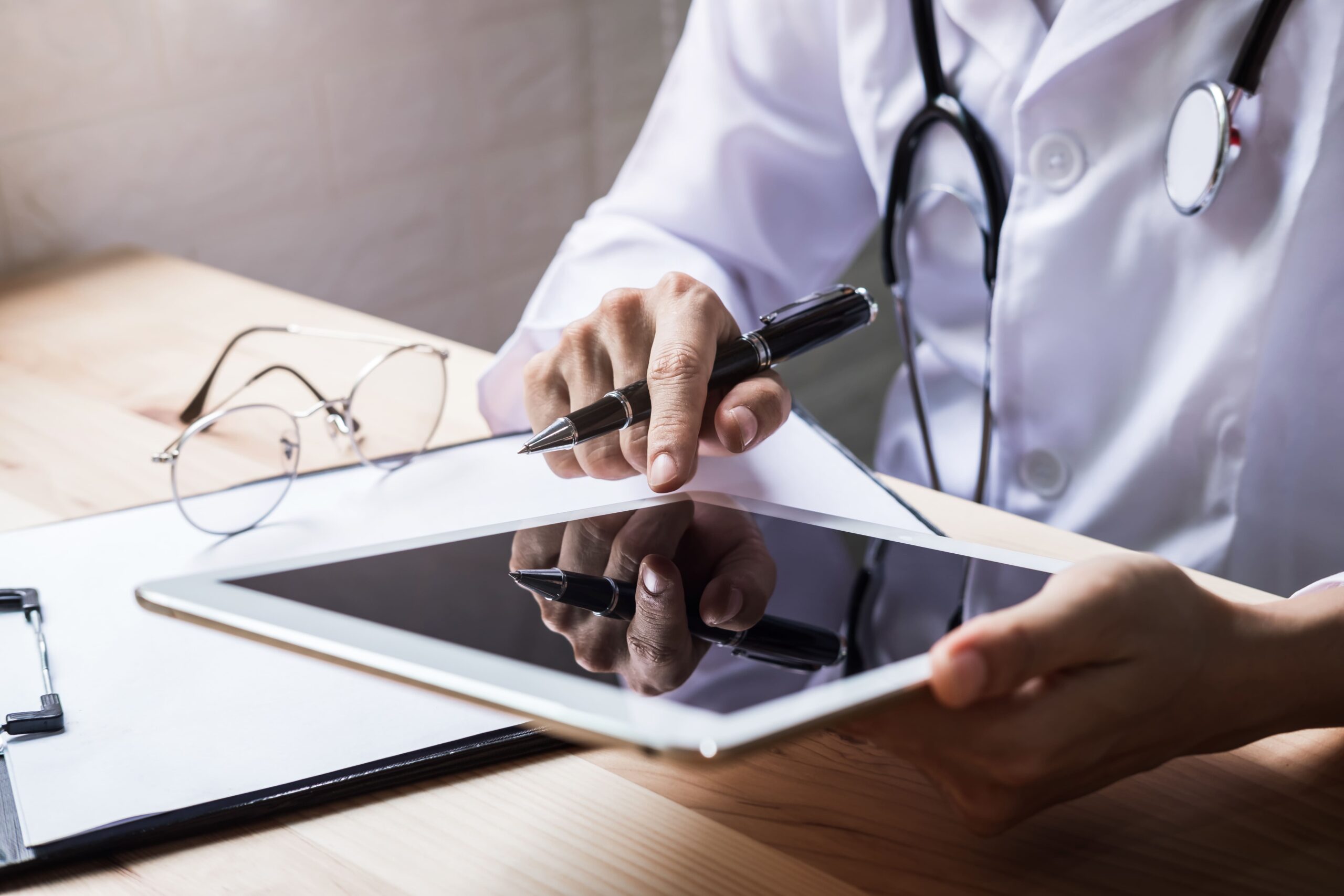
Benefits of AI in medical imaging
Integrating AI into medical imaging brings a wide range of benefits that could transform the field of radiology. Here are some of the most significant advantages:
Enhanced diagnostic accuracy
One of the most significant advantages of AI in medical imaging is its ability to enhance diagnostic accuracy. AI algorithms can analyze images at a speed and precision that surpasses human capability, identifying subtle patterns or abnormalities that radiologists might miss. This is especially vital for the early detection of diseases such as cancer, where even the smallest changes in imaging can be crucial in confirming or ruling out a diagnosis. AI’s ability to process and analyze large datasets also allows it to detect patterns across diverse populations, making it an invaluable tool for identifying rare conditions or monitoring disease progression over time.
Minimization of human error
Radiologists, like all healthcare professionals, are not immune to fatigue, distractions, or the limitations of human perception. AI serves as a secondary layer of analysis, reducing the risk of diagnostic errors. For example, detecting small nodules in lung scans or accurately measuring tumor sizes can be challenging, particularly when faced with a high volume of cases. AI’s consistent ability to identify these anomalies minimizes the chances of missing crucial details, improving diagnostic accuracy and ultimately enhancing patient outcomes.
Increased efficiency and productivity
AI’s automation capabilities significantly enhance the medical imaging process. AI enables radiologists to concentrate on more intricate and critical aspects of patient care by taking over routine tasks like image acquisition, report generation, and scheduling. This is especially important given the rising demand for imaging services, as AI can handle large amounts of data much more quickly than human operators. For instance, AI-driven virtual assistants can create preliminary radiology reports, allowing radiologists to review and refine them instead of starting from scratch. This results in quicker turnaround times for results and lessens the burden on clinicians.
Cost reduction
The financial benefits of AI in medical imaging are significant. By optimizing imaging resources, AI helps reduce the need for repeat imaging, decrease diagnostic errors, and minimize unnecessary tests or procedures. Studies show that AI’s ability to improve diagnostic accuracy could lead to substantial cost savings, especially in emergency departments, where imaging processing and exchange costs are exceptionally high. Additionally, by minimizing human error and accelerating diagnosis times, healthcare facilities can reduce the overall cost of patient care, contributing to the financial sustainability of healthcare systems.
Support for radiologist burnout prevention
Radiologists often face heavy workloads, long hours, and mental fatigue, which can lead to burnout. AI helps alleviate some of this cognitive strain by automating repetitive tasks and assisting with routine image interpretations. This reduces the pressure on radiologists, allowing them to focus on more complex cases, patient consultations, and other critical decision-making tasks. AI can also be a virtual assistant, offering real-time feedback, suggesting potential diagnoses, and helping radiologists make quicker, more informed decisions. This support not only improves efficiency but also promotes the well-being of radiologists, helping prevent burnout.
Advancements in precision medicine
AI’s ability to integrate and analyze extensive datasets—such as imaging, genomics, clinical notes, and lab results—allows for more accurate predictions of individual patient outcomes. For example, AI can detect early-stage diseases that might be overlooked by traditional methods, enabling earlier intervention and treatment. As AI advances, it will further refine its ability to tailor treatments based on a patient’s unique genetic profile and medical history, fostering the growth of personalized medicine. This precision approach can optimize treatments, improve patient outcomes, and reduce the trial-and-error approach to care.
Facilitation of global healthcare access
AI-driven medical imaging technologies can be utilized remotely, facilitating access to high-quality diagnostic tools for healthcare providers in under-resourced regions. By leveraging cloud-based solutions and telemedicine platforms, AI can support healthcare professionals in remote or underserved areas by interpreting medical images, offering consultations, and even making diagnoses. This capability can connect advanced medical centers with rural or low-resource healthcare environments, thereby improving access to timely and accurate diagnoses on a global scale.
Challenges in implementing AI in medical imaging
Despite AI’s significant advantages in medical imaging, several challenges must be overcome to ensure its successful implementation. These include the high costs associated with initial setup, concerns about data privacy, the necessity for regulatory frameworks, and resistance to change among healthcare professionals. Developing effective AI models also requires large volumes of diverse, high-quality data, which can be challenging to gather and standardize. Overcoming these obstacles will necessitate collaboration among technologists, clinicians, regulators, and policymakers.
The future of AI in medical imaging is promising, with the potential to significantly improve diagnostic accuracy, optimize workflows, and reduce healthcare costs. As AI technologies evolve, they are poised to become an integral part of the radiology toolkit, complementing human radiologists and transforming how medical imaging is applied in patient diagnosis and treatment. However, to fully realize this potential, it is crucial to overcome key challenges, such as seamless data integration, system interoperability, and ensuring that AI is used ethically within healthcare settings. By fostering collaboration between the medical, technological, and academic sectors, we can build a future where AI and medical imaging work together to enhance patient care and reshape the healthcare landscape.
References
IGI Global. (2024). Future Medical Imaging. https://www.igi-global.com/book/future-medical-imaging/332796
PostDICOM. (2023, octubre 24). The Future of AI in Medical Imaging. https://www.postdicom.com/en/blog/future-of-ai-in-medical-imaging
Georgieva, M., & Blakely, E. (2024). Artificial intelligence and its role in radiology: Current applications and prospects. Radiology, 302(1), 231114. https://pubs.rsna.org/doi/full/10.1148/radiol.231114
Medical Futurist. (2023, octubre 10). The future of radiology and AI: What we can expect. https://medicalfuturist.com/the-future-of-radiology-and-ai/