Radiomics in oncology: Advancing precision medicine in cancer care
Radiomics has emerged as a transformative tool in oncology, offering new dimensions in precision medicine by extracting complex, quantitative data from medical images. By systematically converting radiological data into mineable, high-dimensional features, radiomics enables a deeper understanding of tumor biology, behavior, and treatment response. This evolving field enhances diagnostic accuracy, prognostic assessments, and clinical decision-making, bridging the gap between imaging technologies and personalized cancer care.
In oncology, radiomic workflows involve a multidisciplinary approach that spans data curation, tumor segmentation, feature extraction, and advanced modeling. When integrated effectively, these processes enable the prediction of clinical outcomes such as treatment response or overall survival, contributing to more individualized therapeutic strategies. By leveraging machine learning and statistical models, radiomics can distinguish benign and malignant lesions, monitor tumor progression, and predict treatment efficacy.
Despite its promise, implementing radiomics in clinical practice presents several challenges, including data heterogeneity, the need for rigorous validation, and the risk of overfitting models. As interest in this field grows, adherence to standardized guidelines and validation protocols will ensure that radiomics advances toward its potential as a cornerstone of precision oncology.
The role of radiomics in oncology
The core principle of radiomics in oncology is that imaging data can reveal detailed information about tumors that may not be visible through traditional diagnostic techniques. By analyzing variations in signal intensities and spatial relationships of pixels in medical images, radiomics can identify patterns linked to tumor heterogeneity, prognosis, and treatment response. These patterns serve as a “virtual biopsy,” providing a more comprehensive view of the tumor than a traditional biopsy, which typically samples only a small portion.
In clinical practice, radiomics applications can be divided into two main categories:
- Classification tasks: Radiomics models classify tumors as benign or malignant, assess tumor stages, and identify genomic status.
- Predictive models: These models predict clinical outcomes, such as overall or disease-free survival, by analyzing the temporal progression of tumors.
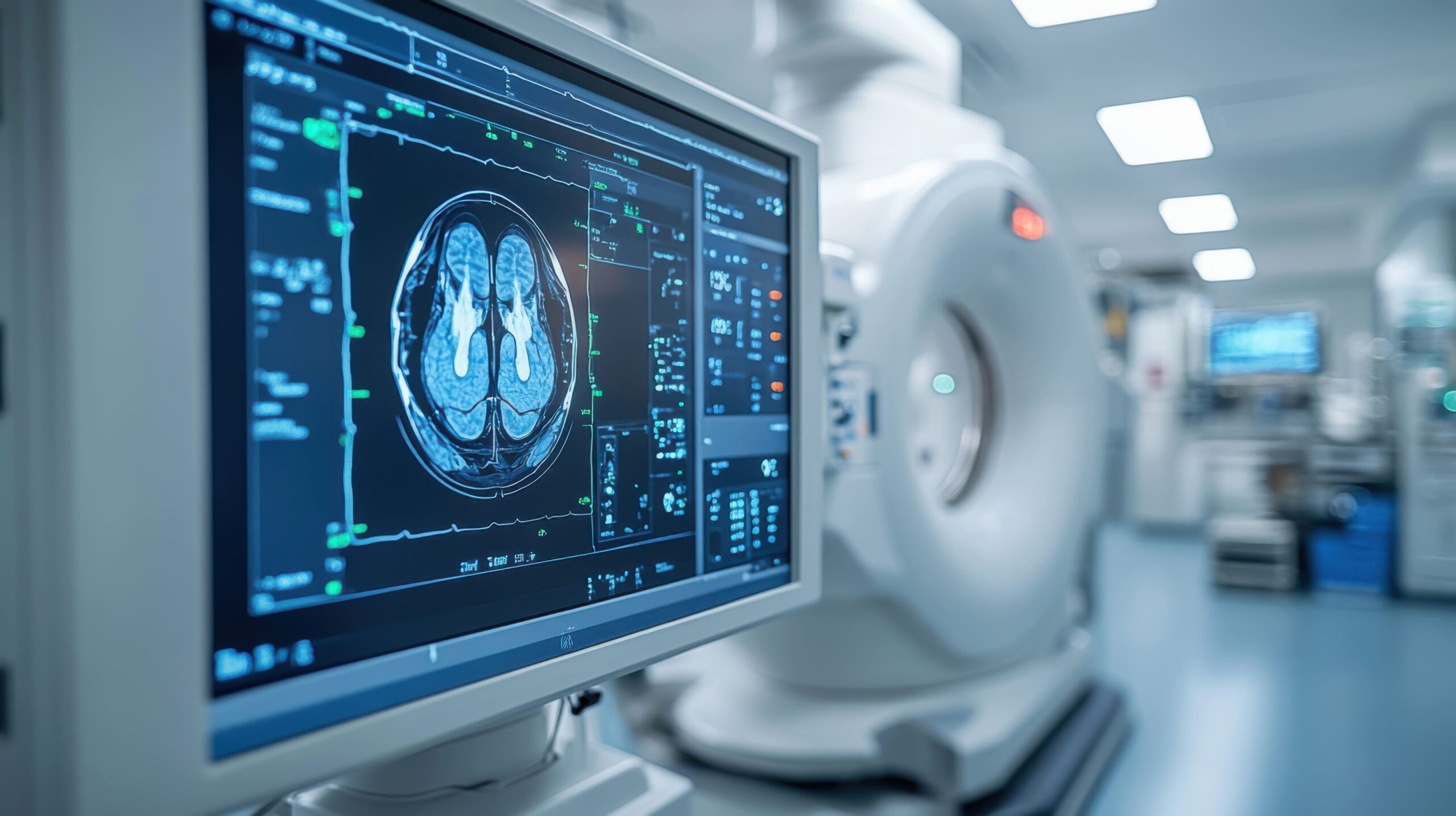
Radiomics software and AI integration
The rapid growth of radiomics in oncology has been supported by the development of advanced radiomics software and radiomics AI tools. These platforms streamline the complex data extraction, feature selection, and model-building processes, crucial for integrating radiomics into routine clinical practice. Radiomics AI enhances the efficiency of these workflows by automating tasks such as tumor segmentation and feature extraction, allowing for faster and more accurate predictions of clinical outcomes.
Several radiomics software applications exist, each offering specialized features. These include:
- MRI radiomics: Particularly effective in analyzing soft tissue tumors, such as breast and lung cancer, MRI radiomics extracts features that describe tissue heterogeneity, essential for understanding tumor aggressiveness and treatment resistance.
- CT radiomics: Often used in solid tumors like lung cancer, CT-based radiomics provides a clear view of the tumor’s structural characteristics.
Radiomics applications in breast and lung cancer
Radiomics breast cancer research focuses on identifying imaging biomarkers that can differentiate between various subtypes of breast tumors, predict responses to chemotherapy, and estimate survival rates. MRI-based radiomics is particularly valuable in this area because it can capture detailed images of soft tissues, providing insights into tumor heterogeneity that can guide treatment decisions.
Similarly, radiomics lung cancer has significantly improved early detection and treatment planning. By analyzing CT scans, radiomics can distinguish between benign nodules and malignant tumors, assess the likelihood of metastasis, and predict patient responses to therapies like immunotherapy or radiation.
Radiomics applications are not limited to breast and lung cancer but extend to various other cancer types, offering valuable insights across different malignancies. In prostate cancer, for instance, radiomics aids in distinguishing between low-risk and aggressive tumors by analyzing MRI features related to tissue density and shape. In colorectal cancer, radiomics can predict the likelihood of metastasis and response to chemotherapy by evaluating the texture and structural characteristics of tumors on CT scans. Additionally, radiomics has shown promise in assessing tumor progression and treatment response in brain tumors by leveraging MRI data to capture subtle changes in tissue heterogeneity.
The radiomics workflow
The radiomics workflow constitutes a highly structured, multi-step process designed to extract and analyze high-dimensional data from medical imaging, facilitating advanced diagnostic and predictive modeling in oncology. This comprehensive approach is critical for deriving robust, clinically actionable insights to enhance precision medicine and optimize oncological outcomes.
- Tumor segmentation: This crucial step defines the region of interest (ROI) from which features will be extracted. Segmentation can be performed on whole tumors, subregions, or peritumoral zones.
- Image preprocessing: Images undergo normalization to ensure they are suitable for feature extraction, reducing variability that could distort the results.
- Feature extraction: Hundreds of quantitative features are extracted from the segmented region, capturing texture, shape, and intensity.
- Model development: Machine learning techniques are used to develop predictive models that link these features to clinical outcomes.
- Validation: Cross-validation techniques, including internal and external datasets, ensure the model reliably predicts outcomes for new patients.
Challenges and future directions of radiomics in oncology
While radiomics in oncology holds immense potential, several challenges must be addressed to realize its full impact. One significant issue is data heterogeneity arising from variations in imaging protocols, equipment, and patient populations. These inconsistencies can undermine the reliability of radiomic models, making it essential to standardize imaging protocols and ensure the availability of large, diverse datasets to enhance model generalizability and robustness.
Another critical challenge is overfitting, where radiomic models perform well on training data but need to generalize more effectively to new, unseen datasets. To mitigate this risk, it is vital to employ rigorous validation methods and feature selection processes that reduce model complexity and enhance its predictive performance across varied clinical settings.
Finally, clinical integration remains a key hurdle. Despite significant technical advancements, incorporating radiomics into routine clinical practice requires further validation and standardization to ensure its utility and reproducibility in real-world scenarios. Establishing clear guidelines and protocols for implementing radiomics in oncology will be crucial for its broader adoption.
As interest in radiomics grows, integrating radiomics AI and machine learning will likely accelerate its adoption, allowing for more efficient data processing and predictive modeling. Additionally, advancements in radiomics software and imaging techniques, such as MRI radiomics, promise to refine the precision of cancer diagnostics and treatment planning, ultimately improving patient outcomes.
References:
Harish, G., Rajiah, P., & Ghosh, A. (2022). Spectrum of imaging findings of primary and secondary cardiac tumors. RadioGraphics, 42(1), 106-123. https://doi.org/10.1148/rg.2021210037
Rahouma, M., Baudo, M., Yahia, S., Kamel, M., Spadaccio, C., Al Ayoubi, M., Elmously, A., Zaitoon, O., & Gaudino, M. (2021). Cardiac tumors: Clinical presentation, diagnosis, and management. Heart, Lung and Circulation, 31(5), 766-778. https://doi.org/10.1016/j.hlc.2021.09.011
Fujimoto, D., Yoshimatsu, Y., Nonaka, M., Tomii, K., Okano, N., & Hirai, T. (2022). Pembrolizumab-induced myocarditis: A Japanese case series and review of the literature. The Oncologist, 27(6), e471-e474. https://doi.org/10.1093/oncolo/oyac073
Gainor, J. F., Raje, N., Huggins, J. T., Yee, J. Y., Alessi, J. P., Benbernou, N., … & Fehrenbacher, L. (2024). A phase II study of CDK4/6 inhibition with abemaciclib in malignant pleural mesothelioma. JCO Precision Oncology, 8, 1-10. https://doi.org/10.1200/PO.24.00155