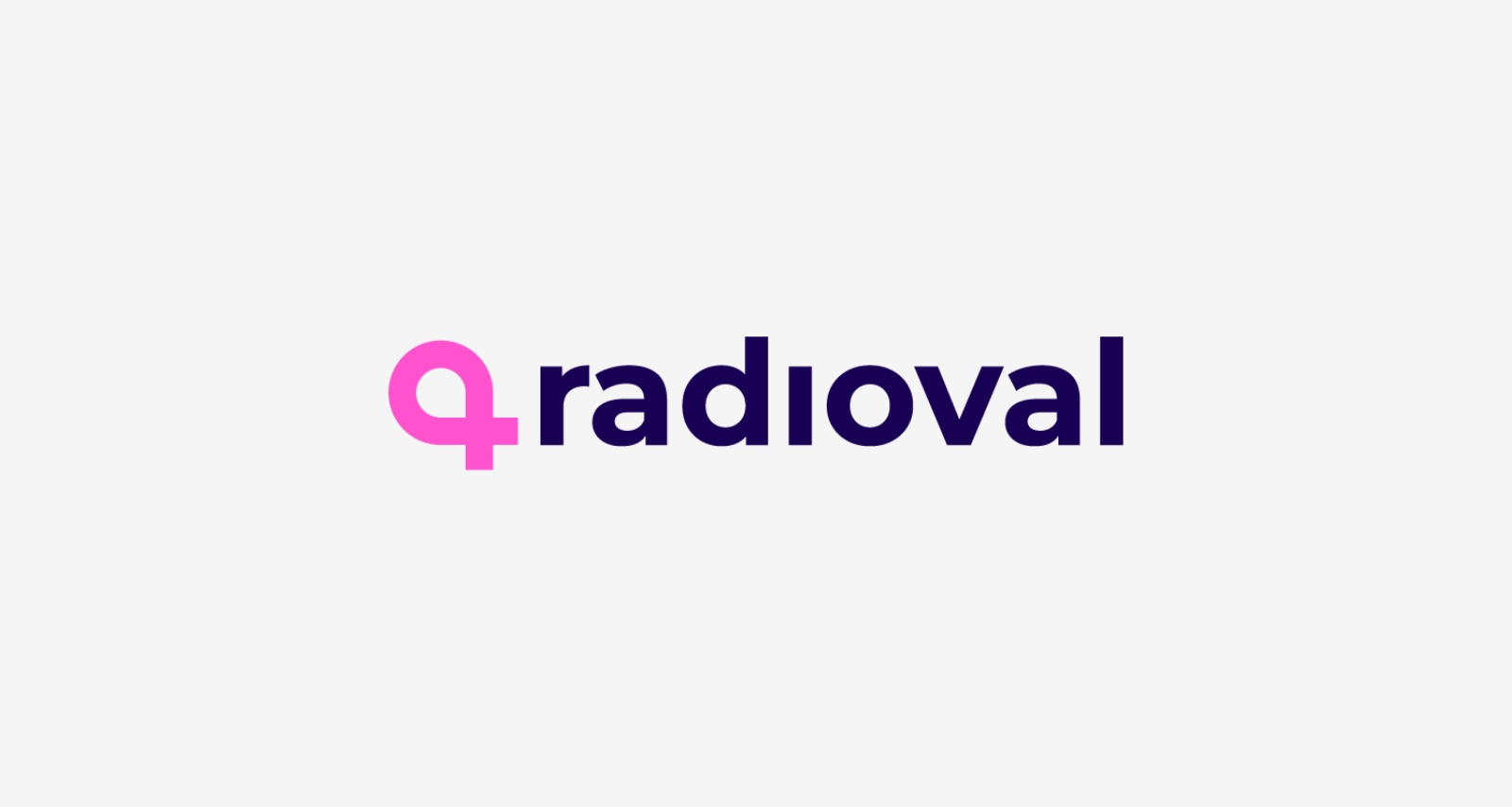
Sometimes critical information cannot be seen through standard visual inspection of medical images, especially in oncology. However, radiomics is a quantitative image analysis method that can reveal the invisible. Utilizing artificial intelligence (AI) analysis techniques, radiomics can quantify visual differences in image intensity, shape, or texture, overcoming the subjective and time-consuming nature of image interpretation. [1].
Nowadays, breast cancer is the most frequent type of cancer worldwide, accounting for almost 30% of all malignancies in women, and current trends show that its incidence is increasing[2]. Given the magnitude of the problem, the urgency for an effective treatment is pressing.
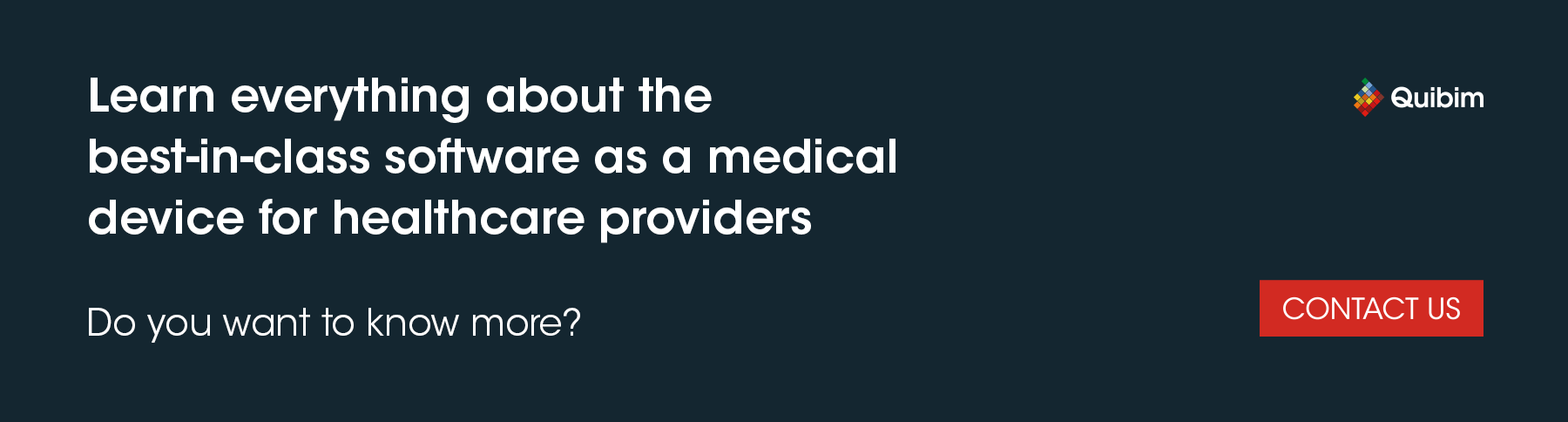
So far, neoadjuvant chemotherapy[3] (NAC) ‘s primary goal was to shrink the tumor size (also known as downstaging) to allow breast-conservation surgery and avoid an even major procedure.
Although NAC has shown the potential to lower mortality in advanced cases, it is associated with a high rate of over-treatment and significant side effects for patients. On the other hand, in lower-income countries, under-treatment has been found due to the high costs of NAC. RadioVal will evaluate the potential of radiomics to predict response to NAC in breast cancer patients with the help of AI, improving patient selection and reducing both over- and under-treatment therapy.
RadioVal is the first multi-dimensional clinical validation of radiomics-based NAC response estimation in breast cancer. It will be validated in 8 clinical centers in Sweden, Austria, Spain, Poland, Croatia, Argentina, Egypt, and Turkey. It can be used large image repositories, tools, and results from 5 previous EU-funded AI4HI network projects.
This will be the first international validation of a radiological AI solution in breast cancer, with a comprehensive and unified methodology that both physicians and patients can trust, and implemented by healthcare centers and other stakeholders, all while adhering to the FUTURE-AI Guidelines[4], to assess Fairness, Universality, Traceability, Usability, Robustness, and Explainability. From the start, the RadioVal project, will adopt a multi-stakeholder approach, considering clinical and healthcare demands and socio-ethical and regulatory concerns.
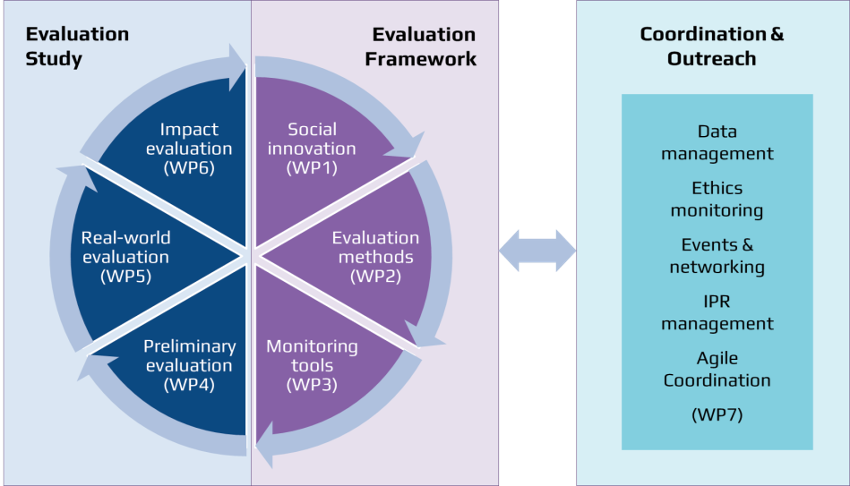
Multi-stakeholder engagement with clinicians and patients, IT and healthcare managers, and ethics and legal experts will guide the implementation of the evaluation methodologies and studies, while continuous feedback from the clinical evaluators will provide empirical data to estimate cost-benefits and impact on clinical guidelines and regulatory approval of the RadioNac tool.
The Universitat De Barcelona, in collaboration with other partners, notably Quibim, is leading this complex project collaboration to evaluate if the tools are technically robust, algorithmically fair, useful, and acceptable in clinical contexts.
Quibim’s Value In RadioVal
Quibim has joined this exciting project to provide a pivotal role in 3 main stages of the RadioVal project.
1. Research, design, and development of novel methods for continuous quality control, monitoring, and traceability of radiomic models.
2. Investigate and develop evaluation procedures for radiomic-based models to predict NAC response in breast cancer.
3. Examine possible exploitation routes and sustainability plans to maximize impact beyond the project.
By introducing a standardized and comprehensive framework for evaluating radiomics AI and developing a traceability tool for transparent monitoring, we hope to build confidence in using this technology in clinical settings. In addition, we will explore the broader implications of implementing radiomics AI, including cost-benefit analysis, ethical considerations, and regulatory implications.
This is a collaborative effort aimed at improving treatment options and unlocking the next generation of medical breakthroughs. Our partnership, utilizing artificial intelligence solutions in large patient datasets, holds the potential to revolutionize diagnosis and patient outcomes and push the boundaries of scientific progress.

This work received funding from the European Union’s Horizon Europe research and innovation programme under Grant Agreement No. 101057699 (RadioVal project).
Views and opinions expressed are however those of the author(s) only and do not necessarily reflect those of the European Union. Neither the European Union nor the granting authority can be held responsible for them.
[1] Herrero Vicent C, et al (2022) Machine Learning Models and Multiparametric Magnetic Resonance Imaging for the Prediction of Pathologic Response to Neoadjuvant Chemotherapy in Breast Cancer. Cancers (Basel). Jul 19;14(14):3508.
[2] European Cancer Information System (October 2020). Breast cancer burden in EU-27. https://ecis.jrc.ec.europa.eu
[3] Sidaway, P., 2020. Neoadjuvant therapy improves pCR rate. Nature Reviews Clinical Oncology, 17(12), pp.718-718.
[4] www.future-ai.eu.