The challenge
Prostate cancer (PCa) is the second most common cancer in men worldwide and the second leading cause of cancer death in men. Most localized PCa represents an indolent disease; however, intermediate- and high-risk patients are more likely to die from prostate cancer and its prognosis is more difficult to predict. For these patients, prognosis remains complex with biochemical recurrence (BCR) marking a significant step towards disease progression and potentially metastases. Therefore, accurate risk stratification and swift diagnosis are imperative to enhance treatment decisions and decrease progression incidences.
The solution
This retrospective, multicenter study, PROVIDENCE, promoted by Johnson & Johnson, S.A., aims to develop an AI model predicting metastatic relapse in 300 intermediate or high-risk localized PCa patients. It utilizes diagnostic medical images including multiparametric MRI (T2-W, DWI, DCE sequences), staging bone scans, and clinical data. This is all consolidated on the QP-Insights® platform. Automatic segmentation of the prostate gland and lesion(s) detection followed by the extraction of quantitative imaging biomarkers (QIBs) is being performed from the MRIs, supplemented by counts quantification from staging bone scans. In conjunction with clinical variables, this data will inform the predictive model, forecasting patient metastatic relapse.
The outcome
The poster presented in European Multidisciplinary Congress on Urological Cancers (EMUC) 2024 congress in Lisbon1, showcased the first interim analysis, where clinical variables of 127 PCa patients were used to predict BCR. Both groups, the BCR (n=38) and no BCR (n=89), despite the imbalance, had a similar clinical distribution. Hence, 9 diagnostic clinical variables were included in the GLMnet model with 5-fold cross-validation for the BCR prediction. The model achieved an AUC of 0.79 (sensibility [se] =0.33, specificity [sp] = 0.39), showed in Figure 1. Surgery as the primary treatment for PCa and clinical stage were identified as the most significant variables influencing the model. In addition, by assessing the SHapley Additive exPlanations (SHAP) values, plotted in Figure 2, it can be concluded that undergoing surgery significantly lowered the risk of BCR, whereas elevated nadir prostate-specific antigen (PSA) levels, older age, and higher International Society of Urological Pathology (ISUP) grades increased the likelihood of BCR during follow-up.
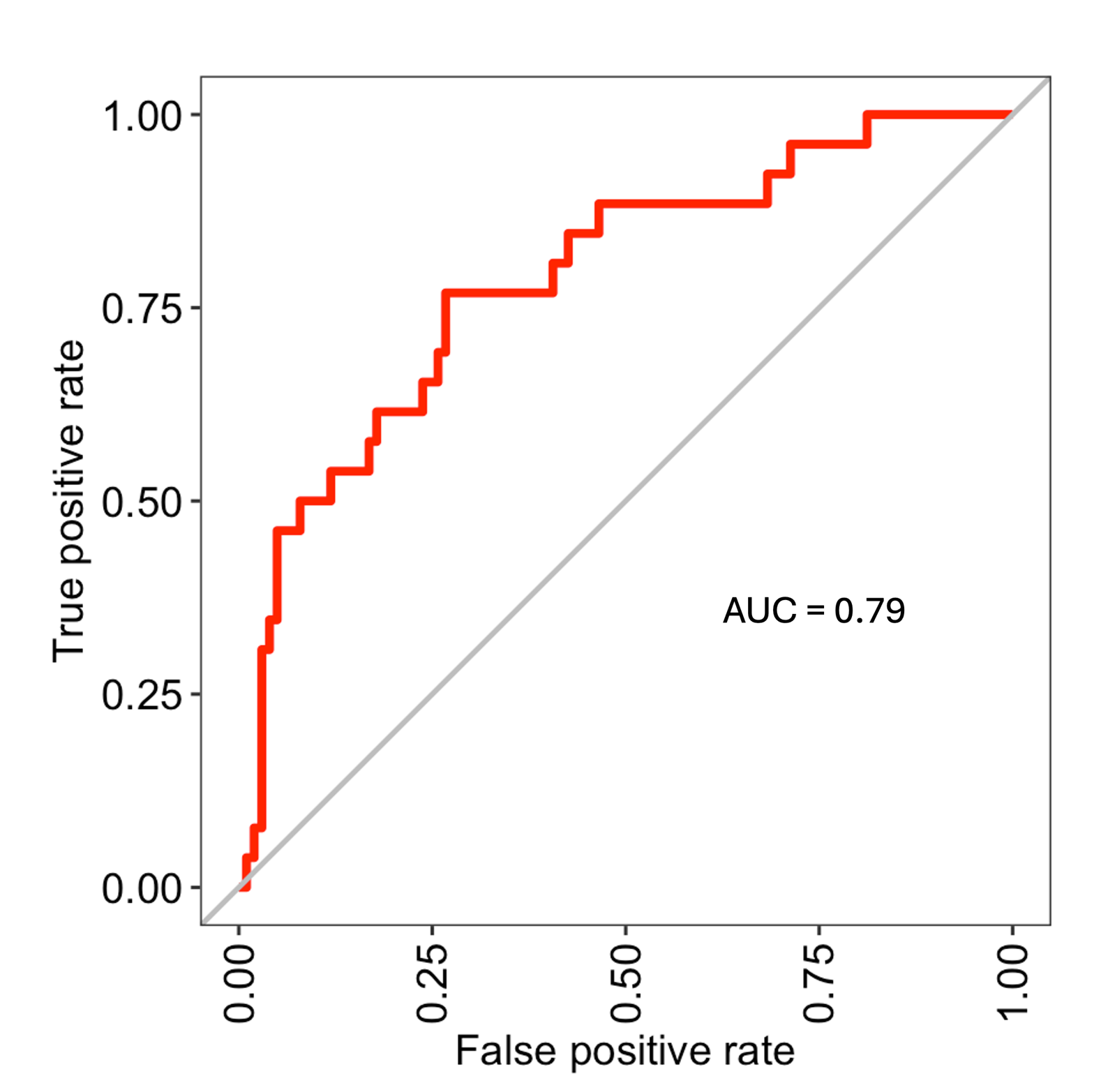
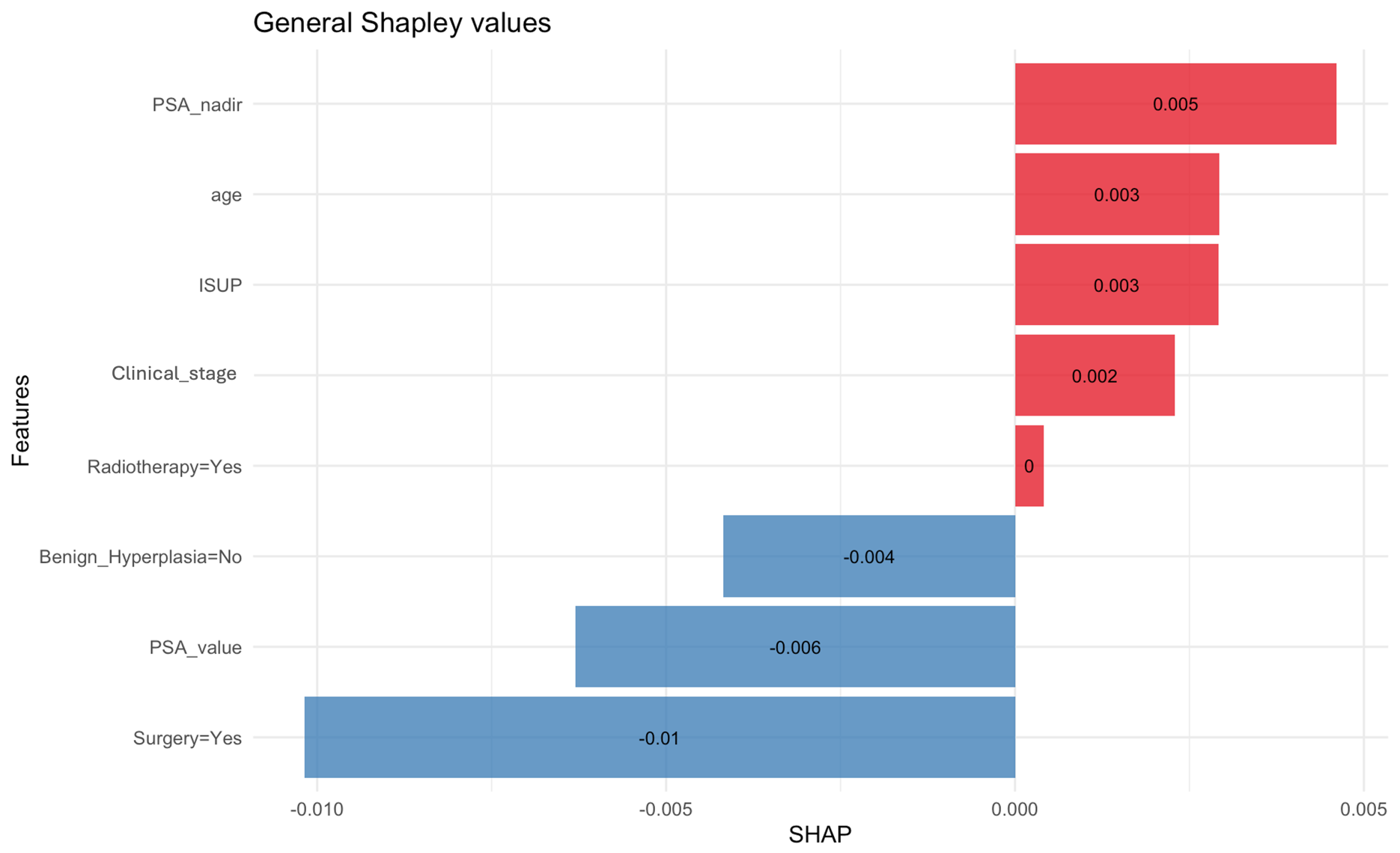
A final analysis that will include a larger cohort of patients, in addition to the incorporation of QIBs extracted from diagnostic images, is expected to yield more definitive insights into the prediction of metastatic risk in localized PCa patients. This would enable non-invasive real-time patient stratification and thereby facilitate the customizing adjuvant therapy and the potential intensification of initial treatments for localized PCa patients diagnosed as intermediate or high risk.
References
-
Conde-Moreno AJ, Torregrosa Andrés A, Gutiérrez Cardo A, et al. P067 An interim analysis of the multi-center retrospective study PROVIDENCE: Artificial intelligence-based models for estimating the risk of biochemical recurrence with clinical data. Eur Urol Open Sci. 2024;69(Suppl 2):80. doi:10.1016/S2666-1683(24)01195-9.
Related case studies
-
Empowering neuroblastoma risk stratification through AI algorithms
Read more
-
Prognostic value of genetic alterations and 18F-FDG PET/CT imaging features in diffuse large B cell lymphoma
Read more
-
CT-based clinical-radiomics model to predict progression and drive clinical applicability in locally advanced head and neck cancer
Read more