The challenge
The client, a life science multinational, was interested in understanding whether AI imaging-based tools could predict whether a non-small cell lung cancer (NSCLC) patient would response to an immunotherapy drug or not and if such a tool could optimize drug development as part of a patient screening process. The generalizability of models to the first dataset would be tested as a follow-on phase.
The possibility of predicting immunotherapy response in advanced NSCLC could be groundbreaking with promising results previously identified. But previous research has identified that the selection of an appropriate extraction method is a major consideration influencing how effective a model is at predictions1,2.
The solution
For the first project phase, we extracted over 5000 radiomics and deep features from thousands of baseline and first on-treatment CT scans across 78 sites and 59 scanner models. We applied in-house harmonization techniques to account for scanner model and other meaningful variabilities that could affect model quality. We then developed many different models based on Overall Survival (OS) and Best Overall Response (BOR) employing different feature combinations and delta radiomic features to develop the most promising models.
For the second project phase we applied our models to a different dataset of NSCLC patients incorporating two treatment arms with different drugs compared to the first phase. The harmonization techniques developed from the first project phase readily transferred to this new dataset and made a very significant reduction in dataset variability. We applied the models from the first project phase and validated specific time-to-event and 12-month OS classification models to analyze the dataset and predict patient response. This allowed us to understand the transferability of models developed on one dataset to another and to validate them.
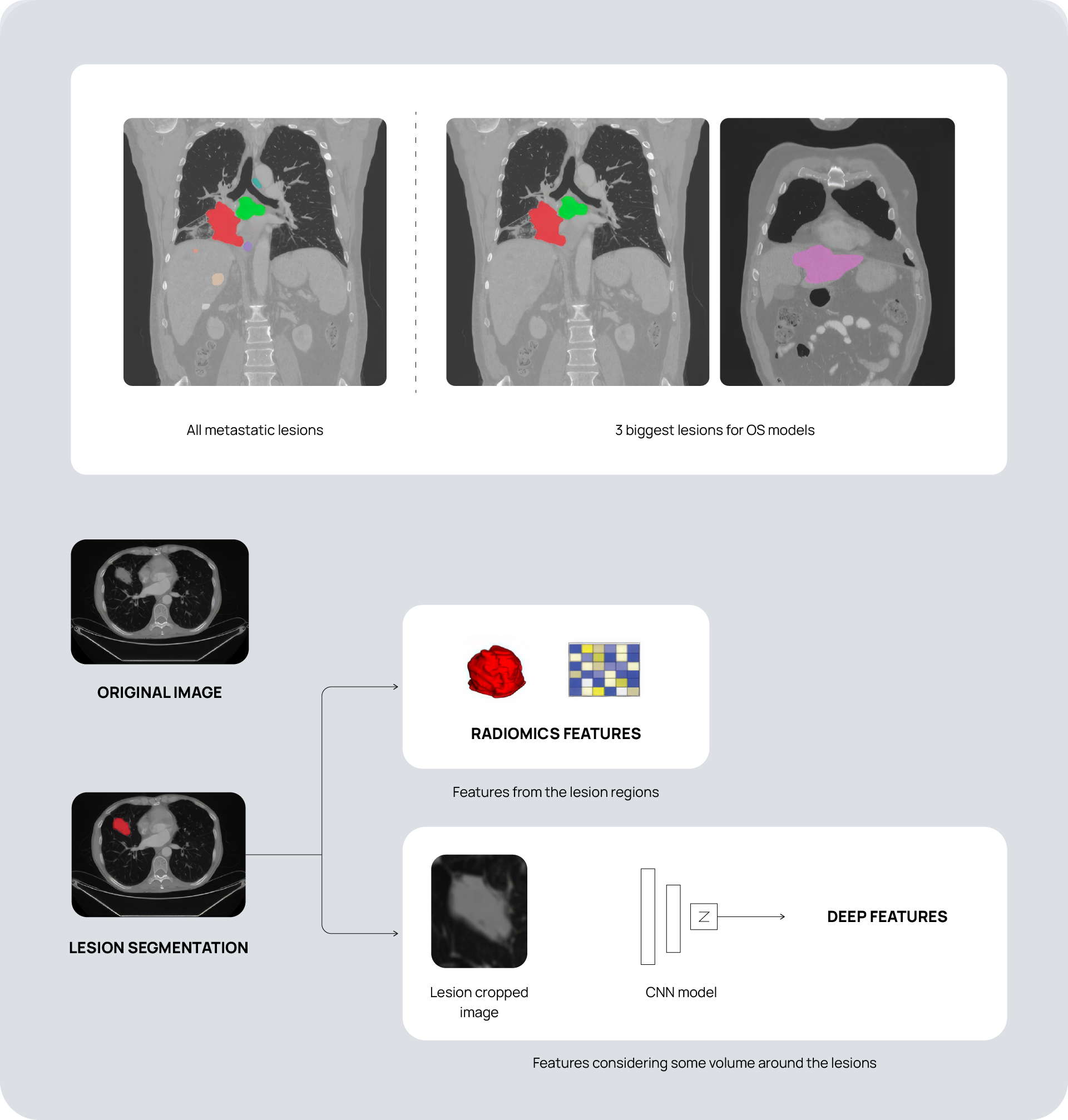
The outcome
Our approach contributed to four principal discoveries:
- Models developed using one dataset can be readily applied to another with good results, showing generalizability and reproducibility.
- The use of radiomics features from baseline and 1st on-treatment CT scans represents a promising non-invasive approach for predicting immunotherapy outcomes in advanced NSCLC patients.
- Impactful radiomics features correlate with intensity and shape pixels linked to tumor heterogeneity and malignancy respectively.
- Laboratory variables contributed to predictions – particularly whether new lesions would appear in the first 60 days.
In general, the best results were obtained by models with the complete set of features (imaging features plus clinical and laboratory data) and combining baseline and 1st on-treatment scan information. No significant difference was observed between models using clinical and laboratory data with radiomics and deep features and models using just radiomics and deep features.
The pre-existing 12-month OS classification model using the features outlined above was applied to the second dataset complete patient cohort with both treatment arms and achieved a C index of 0.718 (C index for first dataset was 0.75 ± 0.05). Even models trained on this second dataset showed positive results when applied to the original dataset, providing evidence that transferability of models to different datasets is favorable. A time-to-event OS model, trained with data from patients of the second dataset, presented a higher accuracy once only included imaging features vs once only included RECIST information (sum of the longest diameter [SLD] at baseline and the SLD ratio between baseline and 1st on-treatment), for the original dataset (C-Index of 0.793 and 0.77, respectively).
As different models incorporated specific features, we found increasingly consistent predictions aligned with the original findings. This underlines the need for the development of rich heterogeneous datasets and for tools and techniques that can leverage the opportunities such data offers.
References
-
Demircioğlu, A. Predictive performance of radiomic models based on features extracted from pretrained deep networks. Insights into Imaging 13, 187 (2022).
-
Urbanos, G. et al. Unleashing deep features and radiomics to enhance best overall response prediction to immunotherapy in advanced non-small cell lung cancer. JCO 42, e20611–e20611 (2024).