The challenge
Breast cancer remains the leading cause of cancer-related deaths among women worldwide, with 2.3 million new cases and 685,000 deaths in 20221. Despite advancements in early detection and screening, predicting treatment responses and disease progression remains a significant challenge2. Oncologists face difficulties in developing treatment plans due to patient and therapy variabilities. However, any empirical approaches often lead to suboptimal patient outcomes and unnecessary toxicities; as an example, patients who might benefit from pre-surgical treatments that reduce mortality such as neoadjuvant chemotherapy (NAC) may go unidentified. Therefore, there is a need for accurate predictive tools that can improve patient outcomes by facilitating treatment personalization while minimizing the risk of side effects.
The solution
Quibim has entered several strategic partnerships to develop radiomics-based models using magnetic resonance imaging (MRI) and fluorine-18 fluorodeoxyglucose positron emission tomography (18F-FDG-PET) imaging for predicting treatment response in breast cancer patients. Specifically, in a published study3 the goal was to perform radiomics analyses to predict pathological complete response (pCR) to NAC, which serves as a surrogate marker for long-term disease-free survival and overall survival. Quibim’s partners supported the collection of MRI and 18F-FDG-PET exams through their hospital networks to train the AI-driven predictive models. These efforts ensure robust and reproducible outcomes across clinical settings:
- Segmentation and Feature Extraction: Quibim’s solution enables the segmentation and extraction of radiomics features from breast tumoral lesions after data harmonization to standardize image quality across centres and scanners.
- Predictive Models for pCR: Quibim offers an imaging-based model for pCR prediction to NAC using radiomics features extracted from MRI and/or 18F-FDG-PET.
This AI-powered tool enhances breast cancer care by providing advanced predictive capabilities for treatment responses while also standardizing and guiding treatment selection in hospitals. By improving patient outcomes, it not only benefits clinical practice but also supports drug marketing strategies effectively.
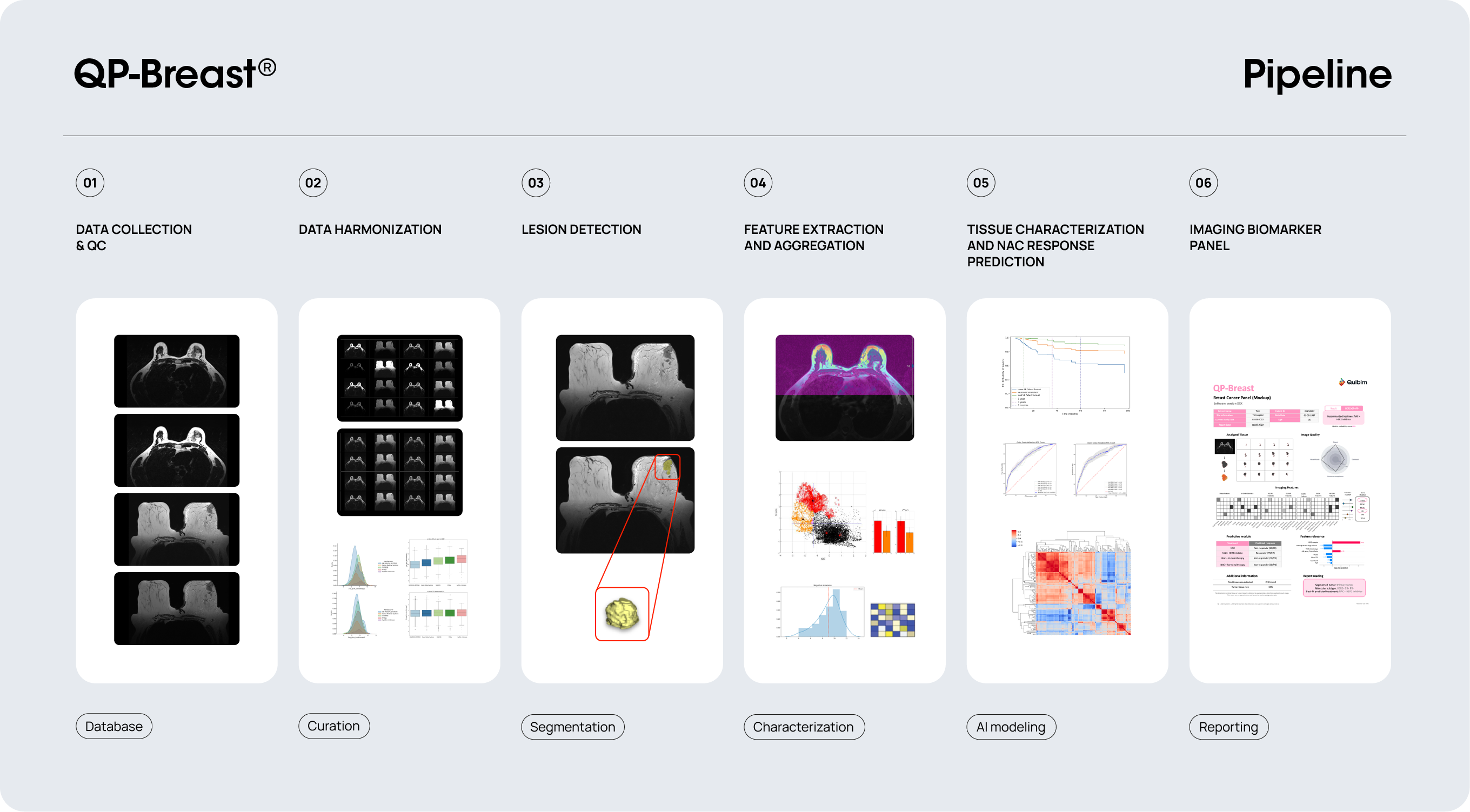
The outcome
These new tools will be implemented and integrated into Quibim’s QP-Breast® product, which is designed to revolutionize breast cancer care by offering advanced predictive capabilities for treatment response and disease progression. This innovative tool will significantly enhance oncologists’ ability to make precise, informed treatment decisions, particularly for aggressive cancer subtypes where there is a critical need for effective decision-support tools. By addressing these gaps, QP-Breast® will represent a major advancement in personalized oncology.
References
- Arnold M, Morgan E, Rumgay H, Mafra A, Singh D, Laversanne M, Vignat J, Gralow JR, Cardoso F, Siesling S, Soerjomataram I. Current and future burden of breast cancer: Global statistics for 2020 and 2040. The Breast 2022, 66, 15 – 23.
- O’Shaughnessy, J.A, Telli, M.L, Bardia, A, Krop, I, McGuire, K. Unmet Needs and Future Direction in HER2+ Breast Cancer, OncLive 2024, 12
- Herrero Vicent, C, Tudela, X, Moreno Ruiz, P, Pedralva, V, Jiménez Pastor, A, Ahicart, D, Rubio Novella, S, Meneu, I, Montes Albuixech, Á, Santamaria, M.Á. et al. Machine Learning Models and Multiparametric Magnetic Resonance Imaging for the Prediction of Pathologic Response to Neoadjuvant Chemotherapy in Breast Cancer. Cancers 2022, 14, 3508.