The challenge
Definitive chemoradiation is currently the standard treatment for LAHNSCC. However, patient responses to the treatment vary, with some experiencing progression within five years of diagnosis. Consequently, there is a pressing need to stratify the risk of progression at diagnosis better, assisting clinicians in making informed treatment decisions. We aim to utilize imaging biomarkers derived from baseline CT scans to classify patients into high or low-risk progression categories.
The solution
In a single-center, retrospective study, baseline CT scans and clinical data from 171 LAHNSCC patients treated with definitive chemoradiation were collected. Tumor segmentation was manually done by Quibim technicians using the Quibim platform under a radiologist’s supervision. Imaging biomarkers were extracted from each lesion, and the data underwent feature reduction. Following a 5-fold cross-validation, we tested several models for their predictive capacity for 5-year progression at diagnosis.
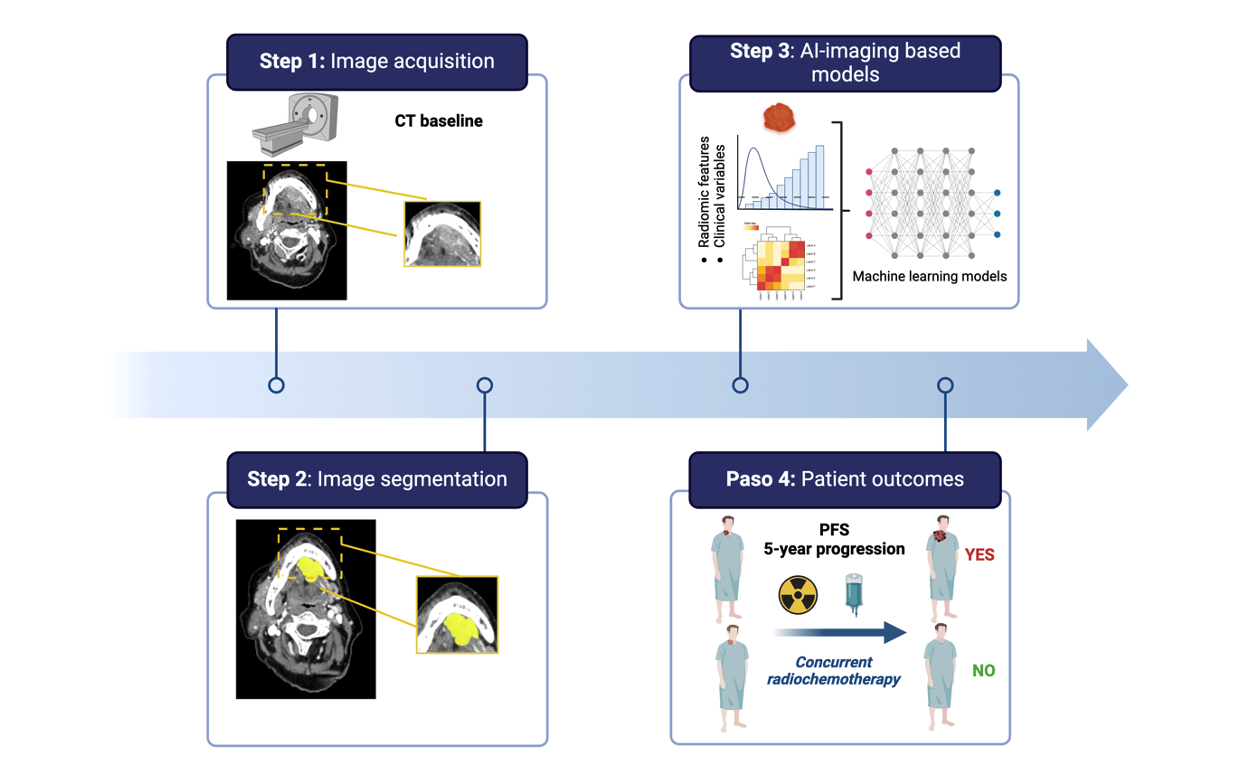
The outcome
In our recently accepted publication in European Radiology, we demonstrate that the extreme gradient boosting model (XGBoost) most effectively predicted 5-year progression, including 12 radiomics features and four clinical variables (primary tumor site [oral cavity], TNM [tumor-nodes-metastasis], age, and smoking), achieving an AUC of 0.74, sensitivity of 0.53, specificity of 0.81 an accuracy of 0.66 on the test set, as shown in Table 1.
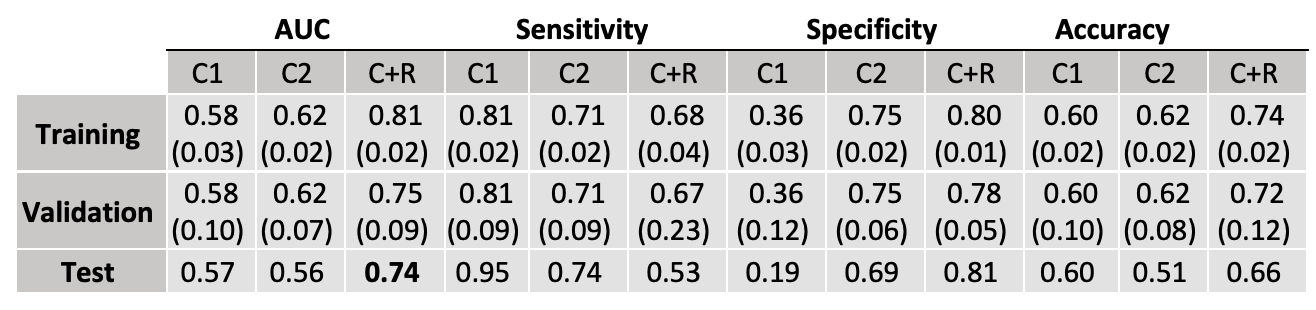
In addition, results obtained in the univariant analysis shows statistically significant differences between progressors and non-progressors in 5 radiomic variables. As displayed in Figure 2, the major axis length plot revealed that the largest diameter of the primary tumor was longer in progressors, while the second-order features revealed a more heterogeneous primary tumor in this same group.
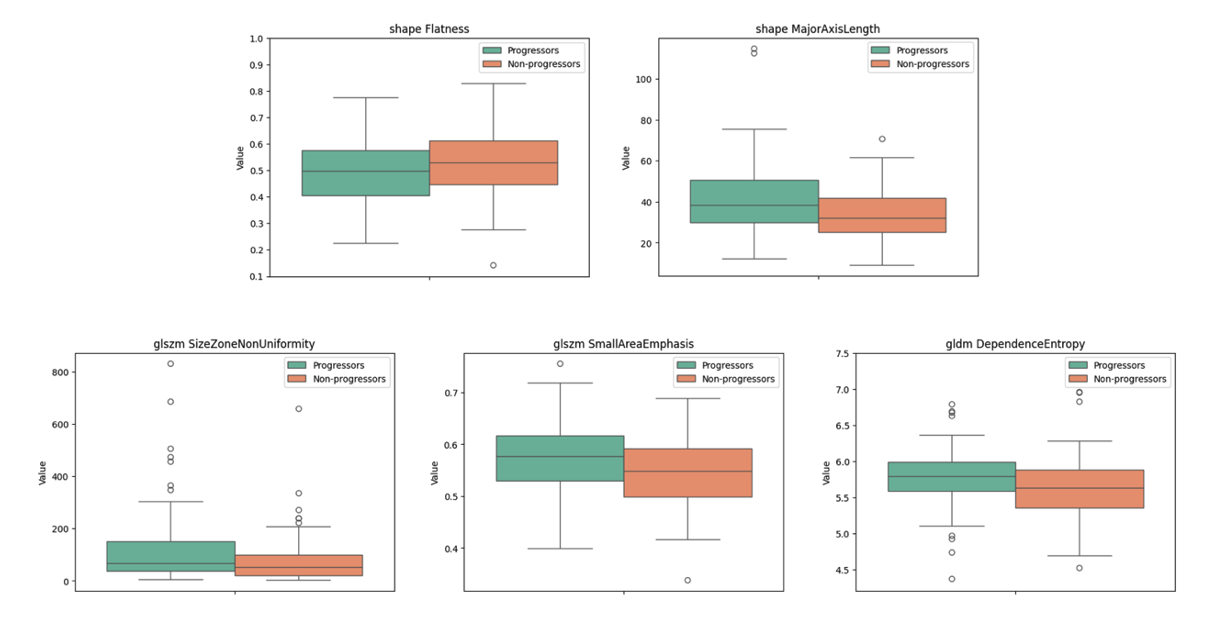
In conclusion, a comprehensive model for LAHNSCC based on CT-radiomics features and including routine clinical variables shows remarkable accuracy in stratifying risk groups, outperforming current clinical models. However, prospective external validation is required to confirm these findings.
Reference:
Bruixola, G., Dualde-Beltrán, D., Jimenez-Pastor, A., Nogué, A., Bellvís, F., Fuster-Matanzo, A., Alfaro-Cervelló, C., Grimalt, N., Salhab-Ibáñez, N., Escorihuela, V., Iglesias, M. E., Maroñas, M., Alberich-Bayarri, Á., Cervantes, A., & Tarazona, N. (in press). CT-based clinical-radiomics model to predict progression and drive clinical applicability in locally advanced head and neck cancer. European Radiology.
Related case studies
-
Empowering neuroblastoma risk stratification through AI algorithms
Read more
-
Prognostic value of genetic alterations and 18F-FDG PET/CT imaging features in diffuse large B cell lymphoma
Read more
-
Managing, storing and analyzing large-scale imaging and multi-omics datasets through QP-Insights® platform
Read more