When you visit any website, it may store or retrieve information on your browser, mostly in the form of cookies. This information might be about you, your preferences or your device and is mainly used to make the site work as you expect it to. The information does not directly identify you but can give you a more personalized web experience. Because we respect your right to privacy, you can choose not to allow some types of cookies. Click on the different category headings to learn more and change our default settings. However, blocking some types of cookies may impact your experience of the site. You can find more information, including a detailed cookie explanation, on our Cookie Policy.
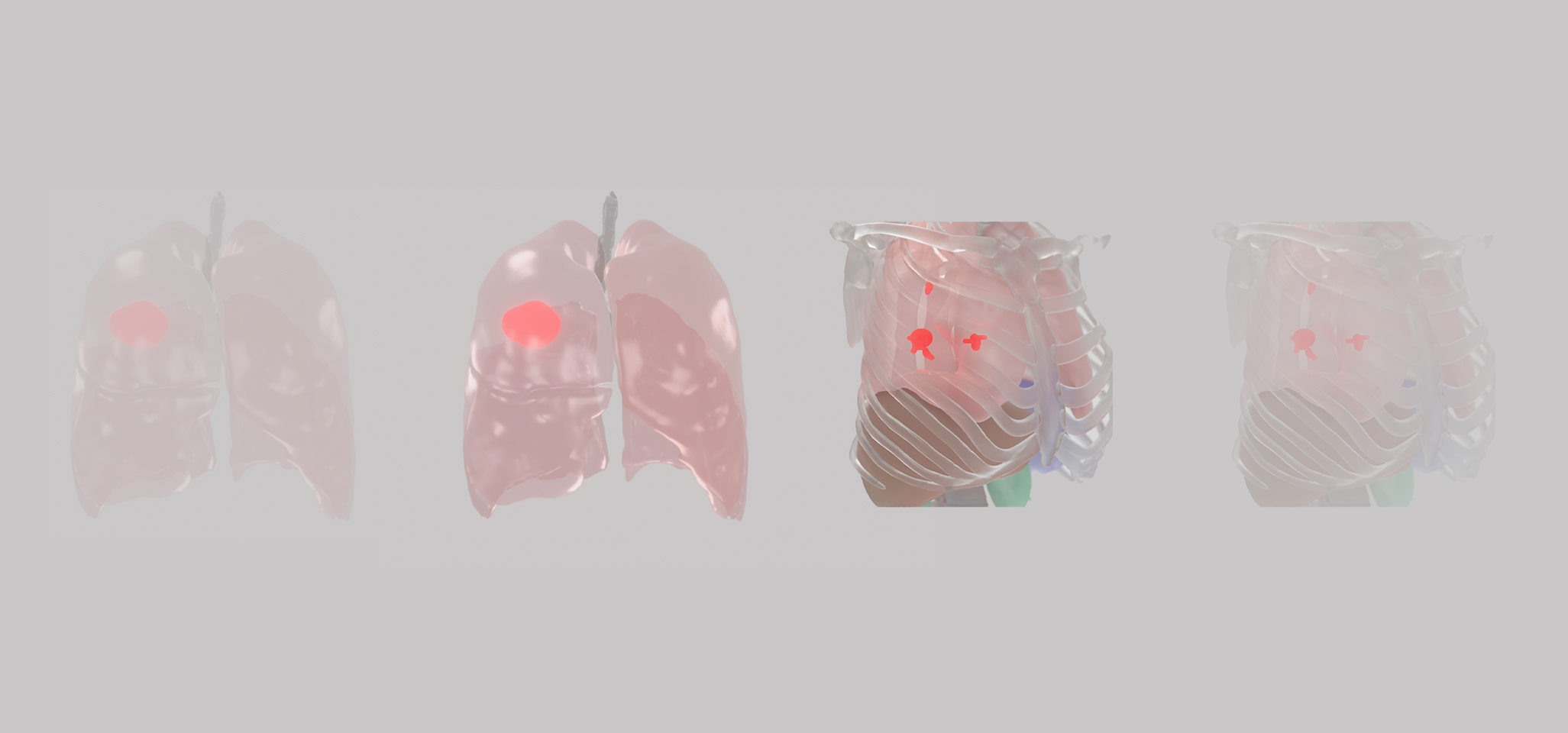
The challenge
A top-tier biopharmaceutical company was interested in incorporating an Artificial Intelligence (AI)-based model that predicts treatment response to immune checkpoint inhibitors in non-small cell lung cancer (NSCLC) using baseline chest CT scans.
Identifying patients more likely to respond to their therapy may improve the design of future clinical studies by maximizing the efficacy of the treatment arm in a specific disease phenotype. In a post-approval scenario, the predictive algorithm would also be useful as a companion diagnostic (CDx) to treat patients likely to benefit from the treatment.
As pay-for-performance reimbursement models grow in the immunotherapy space, it is more important than ever for practitioners to administer the right treatment for the right patient at the right time.
The solution
A large database of more than 1000 chest CT scans from a failed phase-III study was used to extract insights applicable to future studies. The dataset included information about patient outcomes such as treatment response and overall survival. The biopharmaceutical partner engaged in a value-based strategic collaboration with Quibim to design and create an AI model that could predict treatment response based on pre-treatment CT scans.
Quibim performed a feasibility analysis and found a high variability in the image quality across the 70+ sites involved in the study. All images meeting minimum quality requirements were transformed into a standard image quality by GAN-based image harmonization algorithms implemented by Quibim.
After the image quality harmonization of the entire dataset, Quibim developed and validated an AI algorithm based on radiomics, deep features, and deep learning techniques. The resulting algorithm correctly predicted whether or not the patient will respond to immunotherapy in 80 per cent of cases.

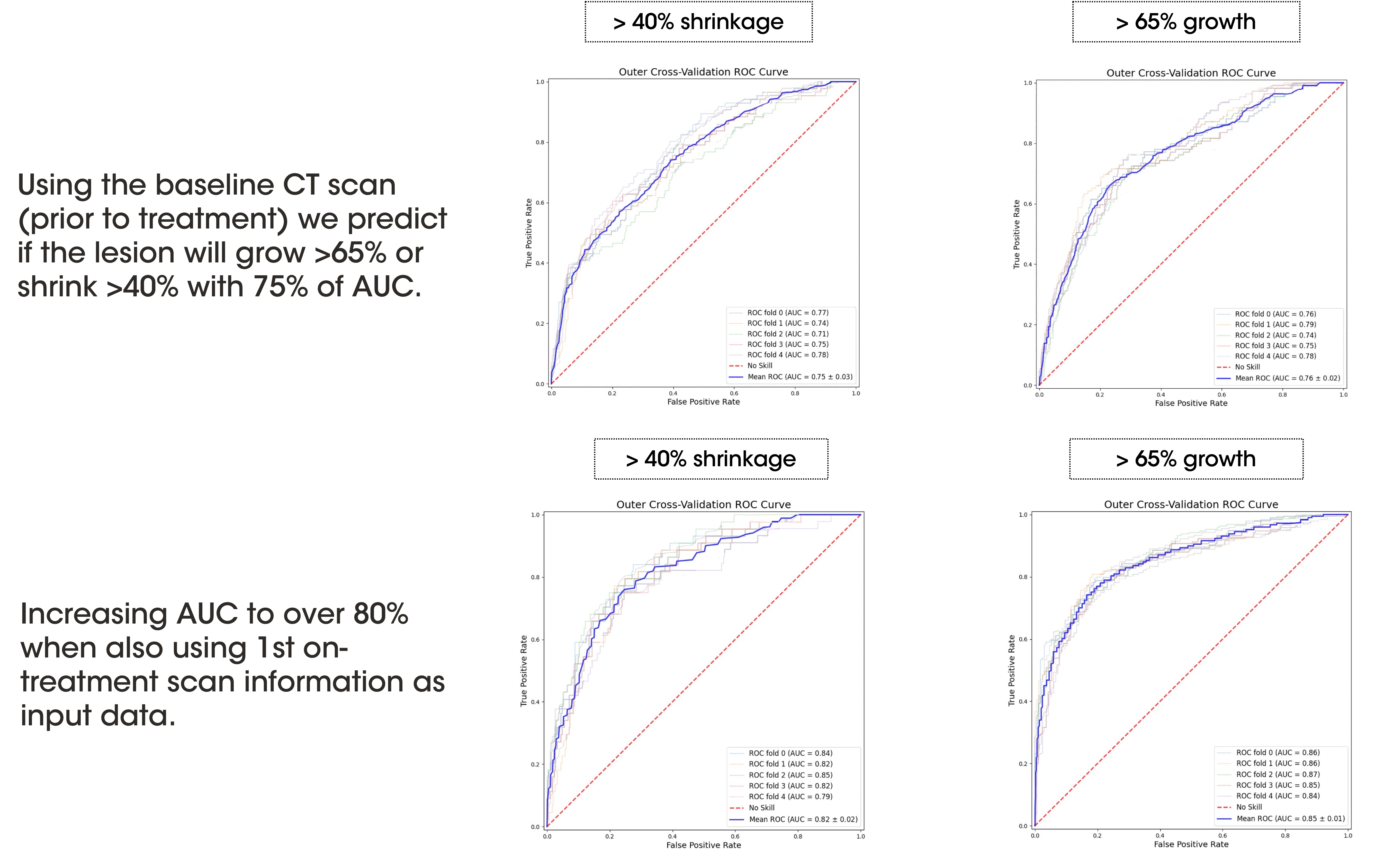
The outcome
What’s in it for the biopharmaceutical partner and Quibim?
- The biopharmaceutical partner plans to license the model and improve the design of future clinical studies in immunotherapy and NSCLC through improved patient selection in the enrolment process, powered by Quibim’s imaging-based AI solution. The algorithm is deployed as a seamless process that can directly communicate with the picture archiving and communications system (PACS) of the hospital for the automated analysis of the CT scans.
- Quibim gained expertise in the creation of NSCLC predictive models and the algorithm generated as a result of the collaboration will be incorporated as a predictive functionality in an upcoming version of QP-Lung® for lung cancer.
Related case studies
-
Empowering neuroblastoma risk stratification through AI algorithms
Read more
-
Prognostic value of genetic alterations and 18F-FDG PET/CT imaging features in diffuse large B cell lymphoma
Read more
-
CT-based clinical-radiomics model to predict progression and drive clinical applicability in locally advanced head and neck cancer
Read more