Patient stratification refers to the process of grouping patients into subcategories based on shared characteristics, such as biomarkers, genetic traits, or disease progression. This approach enables clinicians to personalize treatments, improving outcomes and optimizing resource allocation in healthcare.
Radiomics plays a critical role in advancing patient stratification by extracting valuable information from medical images and converting it into actionable insights for diagnosis, prognosis, and treatment planning.
What is radiomics?
Radiomics refers to the mining of data from medical images, and the subsequent application of such data in enhancing cancer diagnosis, prognostication, and clinical decision support, with the objective of delivering high precision medicine. As a quantitative approach to medical imaging, radiomics techniques are aimed at improving extant data accessible to the clinicians through both advanced and non-intuitive mathematical analysis.
Radiomics in clinical practice
In the field of cancer diagnosis, including prognosis assessment and treatment response evaluation, the application of radiomics is mainly based on the presumption that medical images contain information regarding disease-specific processes that is inaccessible in conventional visual inspection of the images. Using mathematical extraction of spatial signal intensity distribution alongside pixel interrelationships, textural information is quantified by radiomics features through utilization of artificial intelligence-based methods.
Radiomics transforms traditional diagnostic techniques by revealing hidden patterns and biological information that support precision medicine initiatives, including patient stratification biomarkers.
The workflow of radiomics and its application in patient stratification
The premise of radiomics is that imaging data conveys essential information regarding tumor physiology, biology, spatial heterogeneity (habitats), and behavior that might not be apparent to the traditional clinical and radiological interpretation. The workflow of radiomics entails the curation of imaging and clinical data in a stepwise process based on pre-processing of images, segmentation of tumors, extraction of features, development of models, and validation:
- Pre-processing of images
- Tumor segmentation
- Feature extraction
- Model development
- Validation
Radiomics therefore requires the input of professionals drawn from diverse healthcare fields, including imaging specialists, radiologists, as well as data scientists. The derivation of features occurs at multiple or single time points and is applicable to the entire imaging data spectrum.
Radiomic features are categorized into five groups, including shape and size-based features, image intensity histogram descriptors, spatial variation of pixel/voxel intensity descriptors, fractal features, and textures drawn from the filtered images. The information derived from radiomic features can be combined with clinical data to develop effective prediction models targeting treatment selection and prognostic evaluation of clinical outcomes.
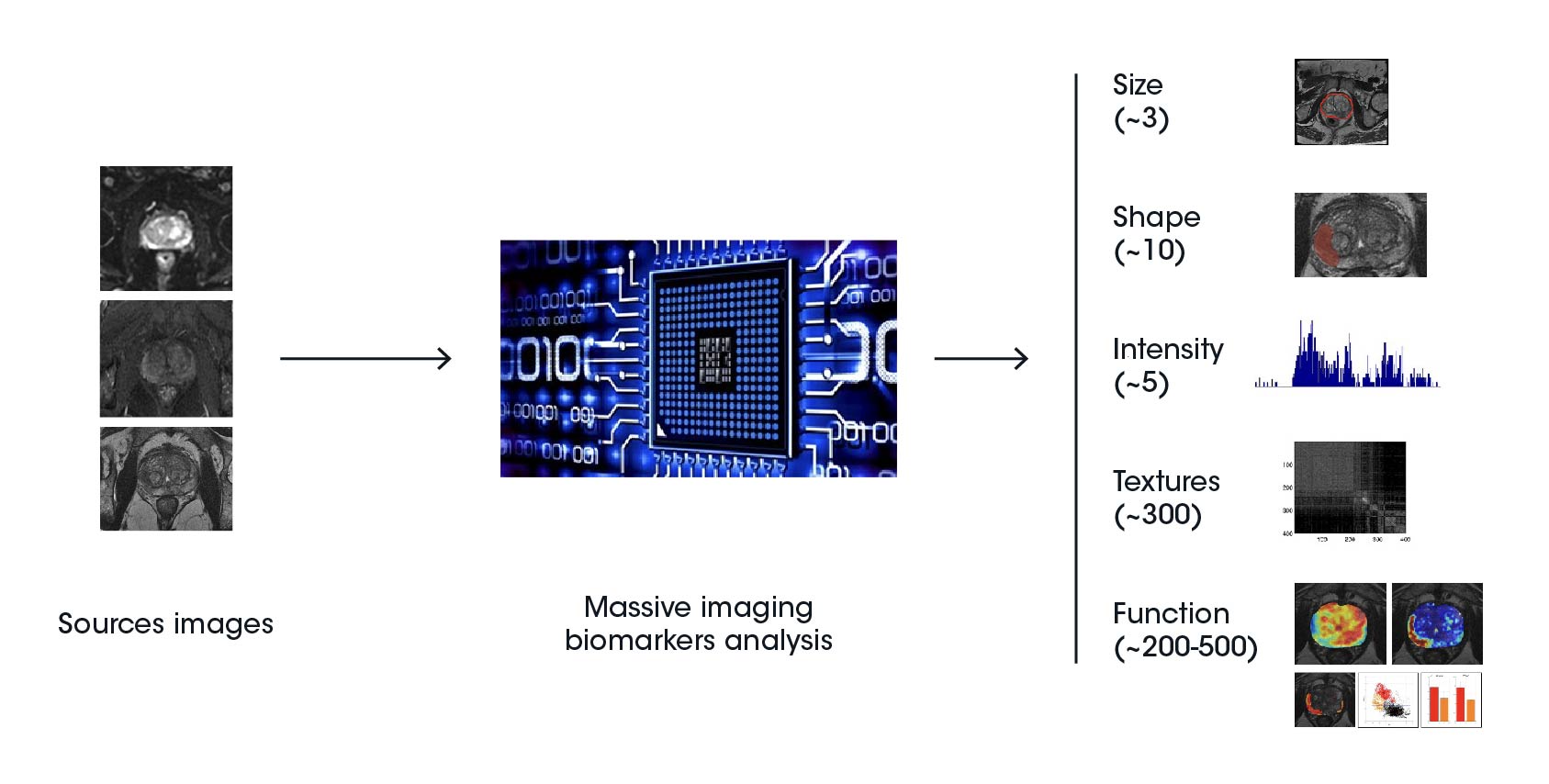
Radiomics features
Patient stratification in oncology
In oncology, radiomics features are used to categorize tasks and predict clinical outcomes using time-to-event evaluation. The classification application entails the division of groups under study into varied categories. In cancer processes, notable instances of classification include malignant versus benign, tumor stages, presence of metastases, and genetic status.
Further, clinical outcomes are employed in the stratification of cancer patients into diverse groups based on aspects of clinical endpoints risk, including disease-free and overall survival, using the time-to-event analysis. Regression models and classifications are frequently applied in modeling molecular markers, genomic signatures, survival prognostication, diagnostic tissue differentiation, and treatment response.
Advances in radiomics
The increase in publicly accessible patient data and open-source artificial intelligence algorithms has led to the development of new multivariate prognostic and diagnostic imaging biomarkers. These biomarkers can also incorporate clinical variables and other multi-omic features in the prediction of outcomes, risk stratification, and the planning of precision cancer treatment.
By integrating radiomics into patient stratification, clinicians can refine their approach to personalized medicine, optimizing care plans based on patient-specific data.
How radiomics companies like Quibim are changing patient stratification
Quibim, a leading radiomics company, is pioneering the development of AI-powered tools to extract imaging biomarkers and integrate them into precision medicine workflows. For instance, Quibim’s contributions to the PRIMAGE project demonstrate how imaging data and clinical data can be combined to improve patient stratification in oncology.
By focusing on digital biomarkers and imaging-based companion diagnostics, Quibim helps clinicians better understand tumor heterogeneity and design targeted therapies for improved patient outcomes.
Radiomics and clinical biomarkers for patient stratification
Clinical biomarkers and companion diagnostics (CDx) allow to find different patient phenotypes as a way of patient stratification. Until now, the development of new biomarkers and CDx has been mainly focused on techniques analyzing real biological samples, such as in situ hybridization, immunohistochemistry, or next-generation sequencing. Indeed, there is only one companion diagnostic in imaging approved by FDA and is related to liver iron concentration quantification.
Radiomic approaches to patient stratification are the next level for the creation of digital biomarkers and imaging-based CDx. The characteristics of tissues and lesions extracted from imaging reflect their architectural heterogeneity, irregularity, and cell habitats, which are factors related to clinical endpoints such as relapse, progression, and survival in existing evidence.
These developments in the imaging field will also help better design new clinical studies and aid in the development of targeted therapies that optimize interventions to an individual patient, thereby increasing success in treating cancer. Radiomics-based patient stratification expedites the translation of clinical and biomedical research outcomes for better care.
Quibim Dataminer adapted for the PRIMAGE project to show, together with the imaging data and the patients’ clinical data.
Quibim Dataminer adapted for the PRIMAGE project to show, together with the imaging data and the patients’ clinical data.